INTRODUCTION
AI is defined and distinguished from other technologies due to its capabilities in terms of data analysis and the creation of outputs. Prime examples are prediction and communication. As such, AI uses for customer-centric services and customer retention create more and better business value. However, its development can be cumbersome, and the result can fail to meet the expectations. This may happen if you don’t know what your problem is, you don’t set the stakeholders’ expectations right, and your data is not ready. Here are three questions you should ask to ensure that AI is what you need and that you are prepared to use it.
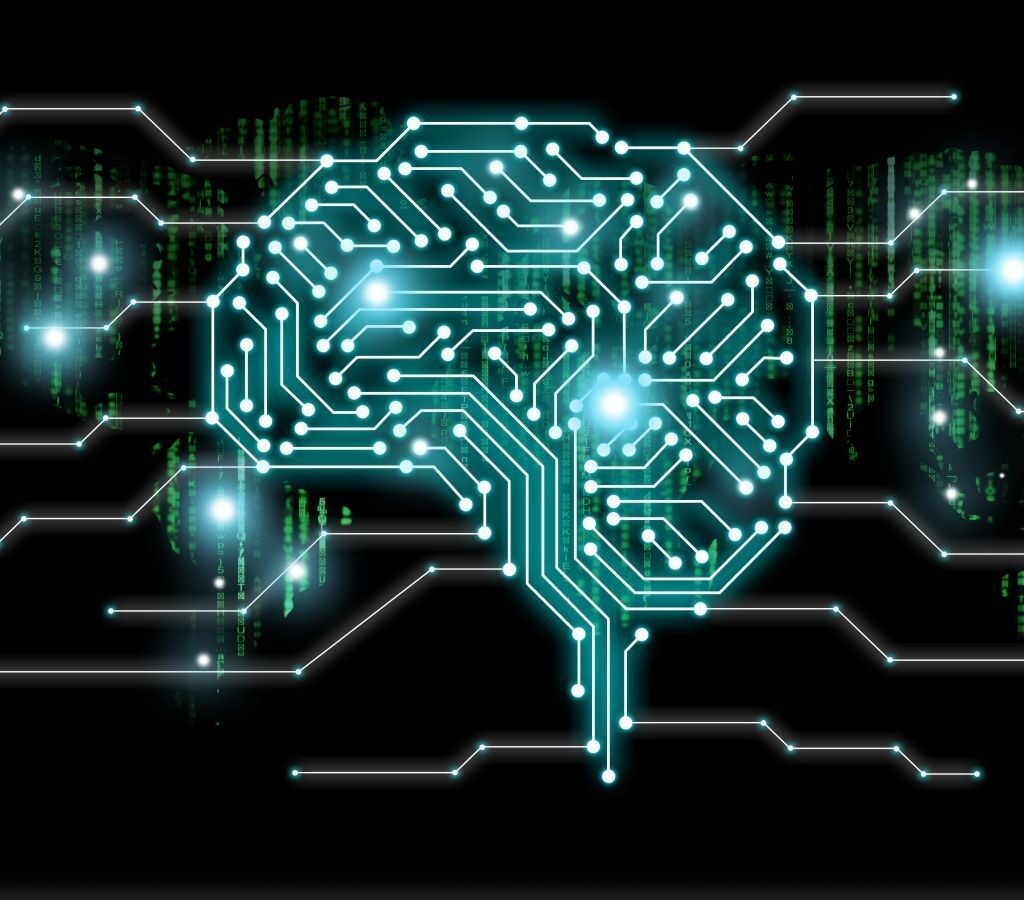
What problem do you expect AI to solve and how the right data can help you?
AI’s capabilities like communication and reasoning are used to solve a range of problems related to more customer-centric support, better predictions of customer churn, and personalization of content like ads. Despite concrete use cases, the hype on AI is still high, and it seems one cannot innovate without it. However, if the problem is not framed in the right way, AI might just be a loosely sewed patch. This can create false expectations and eventually make AI useless for achieving a company’s objective. Besides this, if data is not ready, even a correct framing of the problem with reasonable expectations is not enough. Conversational agents are fit to solve the problem of low customer satisfaction but developing a chatbot expecting it to take care of the full customer support may create the wrong expectations. A conversational agent can provide good answers to repetitive, tedious questions that occupy most of a human agent’s time but there will always be cases that are best addressed by a human. Besides this, if data on customers’ questions and interaction with a prototype of the AI system is lacking, there is still a chance that the chatbot will not meet the expectations.
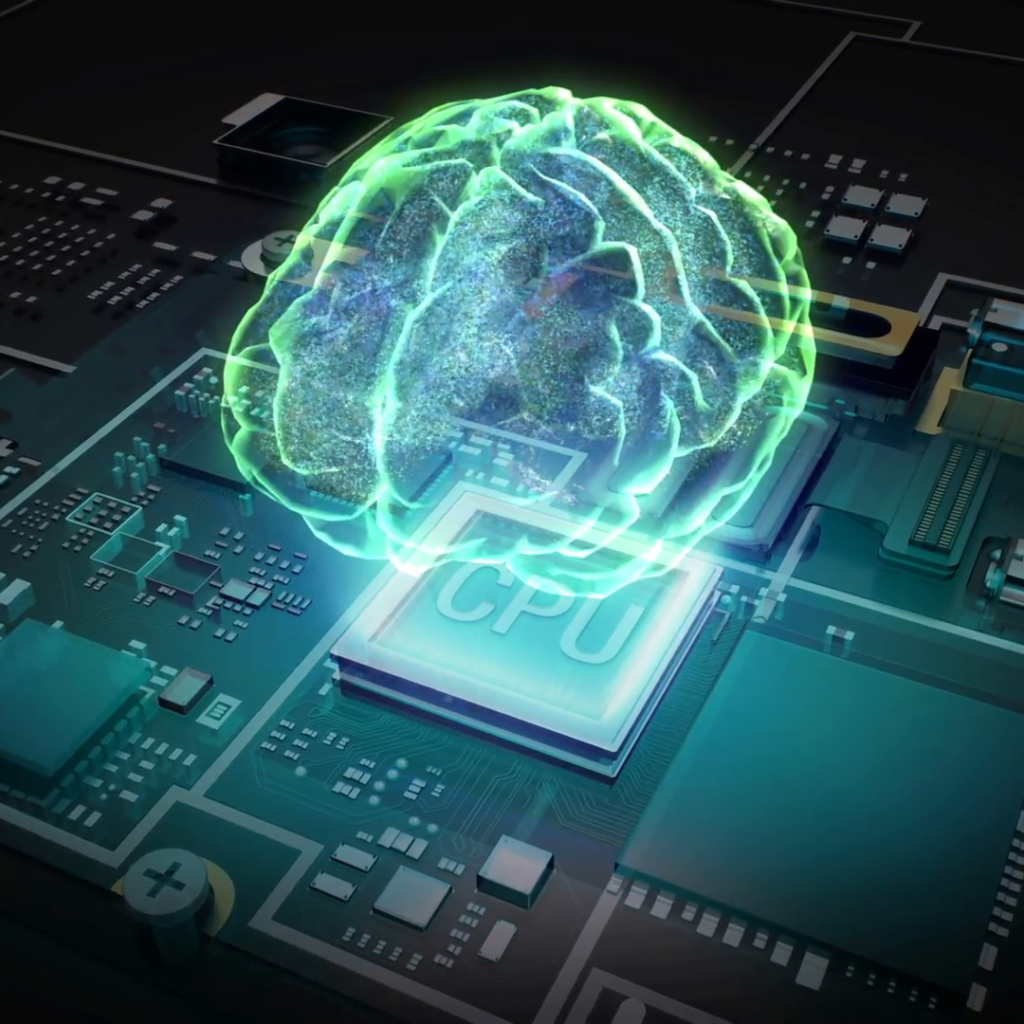
CONCLUSION
Artificial Intelligence capabilities are consolidated through a range of concrete use cases. Conversational agents and customer churn predictions are prime examples. However, there is still much hype around AI that sometimes innovation seems impossible without it. This is not true! AI is a driver of innovation when it is fit-for-purpose. Framing your company’s problem is the key to setting the expectations right, allowing your teams to implement AI tools in their workflow effectively. The correct data must be available. Ask yourself what problem you are trying to solve and if AI’s capabilities can help you and how -what part of the problem can they solve? Start an open discussion with the stakeholders and set shared expectations on the objectives -what can you achieve with AI, and how will it support your processes? Is there any change needed in the team’s workflow? Make sure that you know your data -what data do you have? How are your databases structured? What data do you need for a well-suited and “alive” algorithm?